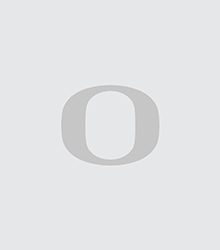
Cengiz Zopluoglu
Biography
Cengiz Zopluoglu is an Associate Professor in the Department of Special Education and Clinical Sciences at the University of Oregon. His research interests are centered around item response theory, psychometrics, latent variable modeling, and integrating machine learning methods into educational measurement. Dr. Zopluoglu has published and contributed numerous articles on psychometrics and statistical methods and their applications in educational and behavioral sciences. Dr. Zopluoglu has taught advanced courses on psychometrics and statistical methodology, including General Linear Models, Item Response Theory, Measurement and Psychometric Theory, Categorical Data Analysis, and Data Analysis with R in Educational and Behavioral Research.
Education
PhD, 2013, University of Minnesota, Twin Cities, MN
Major: Educational Psychology (Quantitative Methods in Education)
MA, 2009, University of Minnesota, Twin Cities, MN
Major: Educational Psychology (Quantitative Methods in Education)
BA, 2005, Abant Izzet Baysal University, Bolu, Turkey
Major: Mathematics Education (K-8)
Honors and Awards
2023 Top Cited Article in Educational Measurement: Issues and Practice, among work published in an issue between Jan 1, 2021 and Dec 15, 2022
2021 National Institute of Justice, Recidivism Forecasting Challenge, 3rd place in Large Team Category
2017 Top 5 Most Cited Papers in Journal of School Psychology 2014-2016
2013 Graduate Student Research Award, University of Minnesota
2007 – 2013 Fellowship for Graduate Education in the U.S., Ministry of National Education, Turkey
Publications
* The following is a list of methodological publications in the past five years.
Zopluoglu, C. & Lockwood, JR (2024). A comparative study of item response theory models for mixed discrete-continuous responses. Journal of Intelligence, 12(3), 26. https://doi.org/10.3390/jintelligence12030026
Kasli, M., Zopluoglu, C., & Toton, S. (2023). A Deterministic Gated Lognormal Response Time Model to Identify Examinees with Item Preknowledge. Journal of Educational Measurement, 60(1), 148-169. https://doi.org/10.1111/jedm.12340
Wang J., Combs T., Zopluoglu C. (2022). Fitting hyperbolic cosine unfolding models using the Stan Language. Journal of Applied Measurement, 23(1/2), 61-73.
Zopluoglu, C., Kasli, M., & Toton, S. (2021). The Effect of Item Preknowledge on Response Time: Analysis of Two Datasets Using the Multiple-Group Lognormal Response Time Model with a Gating Mechanism. Educational Measurement: Issues and Practices, 40(3), 42-51. https://doi.org/10.1111/emip.12428
Park, S.E., Ahn, S., & Zopluoglu, C. (2021). Differential item functioning effect size from the multigroup confirmatory factor analysis for a meta-analysis: A simulation study.Educational and Psychological Measurement,81(1), 182-199. https://doi.org/10.1177/0013164420925885
Zopluoglu, C. (2020). A finite mixture item response theory model for continuous measurement outcomes. Educational and Psychological Measurement, 80(2), 346–364.https://doi.org/10.1177/0013164419856663
Zopluoglu, C. (2019). Computation of response similarity index M4 in R under the dichotomous and nominal item response models. International Journal of Assessment Tools in Education, 6 (5), 1-19. https://doi.org/10.21449/ijate.527299
Zopluoglu, C. (2019). Detecting examinees with item preknowledge in large-scale testing using Extreme Gradient Boosting (XGBoost). Educational and Psychological Measurement, 79(5), 931–961. https://doi.org/10.1177/0013164419839439